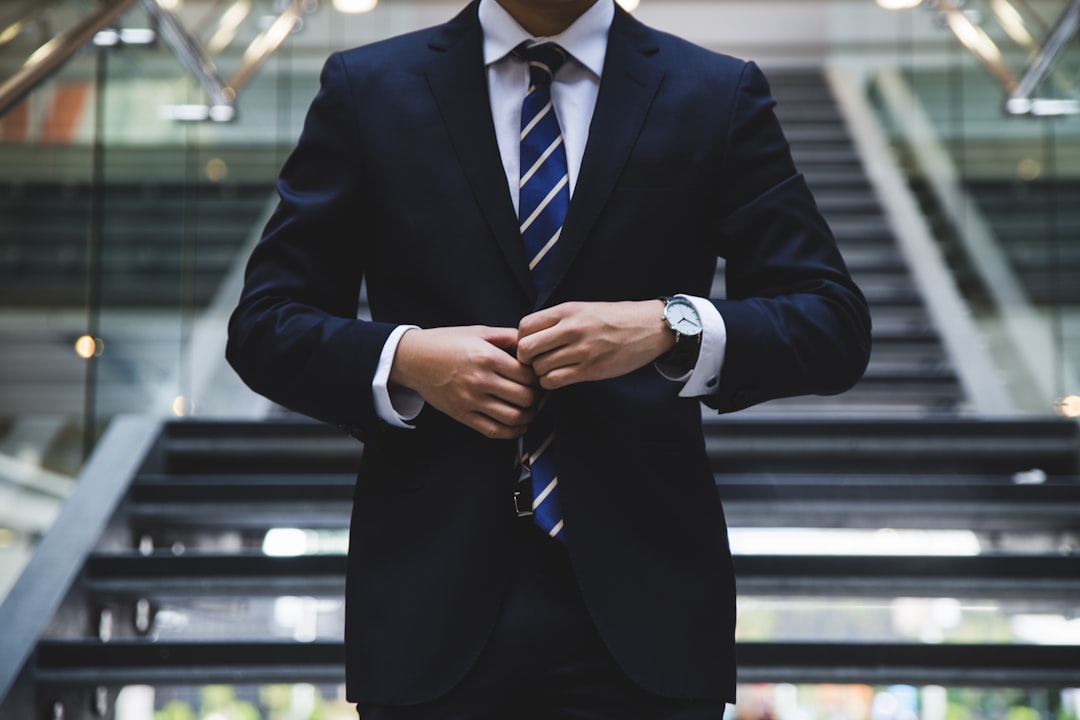
How to Use Econometrics in Research: A Comprehensive Guide
# Introduction. Econometrics is a branch of economics that applies statistical techniques to analyze economic data and test hypotheses. As an evolving field, it plays a crucial role in informing policy decisions and understanding complex economic scenarios. This blog post aims to provide a step-by-step guide on how to effectively use econometrics in research, whether you are a seasoned economist or a novice exploring this fascinating discipline. # Understanding Econometrics: The Foundation of Your Research. Before diving into methods and applications, it's essential to grasp the foundational concepts of econometrics. Econometrics focuses on quantifying economic phenomena through mathematical models. This section will cover the three main types of econometric models: 1. **Descriptive Econometrics**: Often the first step, it involves summarizing data to reveal patterns or trends. 2. **Inferential Econometrics**: This model helps in making predictions or inferences about a population based on a sample. 3. **Causal Econometrics**: A more advanced form, causal econometrics aims to establish relationships between variables, allowing researchers to determine cause-and-effect dynamics. Understanding these types lays the groundwork for utilizing econometric methods effectively in your research. # The Role of Data in Econometric Analysis. Data is the lifeblood of econometric analysis. Whether you’re dealing with cross-sectional, time series, or panel data, the quality and appropriateness of your dataset can significantly impact your findings. Here are some critical steps regarding data: - **Data Collection**: Gather data from reliable sources, ensuring both the relevance and credibility of your dataset. Use reputable databases, government reports, or academic publications. - **Data Preparation**: Clean your data by handling missing values, outliers, and verifying accuracy. Proper preparation sets a solid foundation for your analysis and prevents erroneous conclusions. - **Data Exploration**: Engage in exploratory data analysis (EDA) to identify relationships, distribution, and trends within your data before applying econometric models. # Selecting the Right Econometric Model. Once your data is prepared, selecting the appropriate econometric model is vital. The choice can determine the reliability of your results. Here's how to navigate the decision-making process: - **Identify your Research Question**: Is it descriptive, inferential, or causal? - **Assess Variable Types**: Understand whether your dependent and independent variables are continuous, categorical, or spatial. This will significantly influence your choice of regression methods. - **Choose between Linear and Non-Linear Models**: Linear models are simpler and easier to interpret but may oversimplify relationships in certain datasets. Non-linear models provide a more nuanced understanding but demand more rigorous interpretation. Using these principles will guide you to the most appropriate econometric model for your research question. # Interpreting Econometric Results: Making Sense of the Output. Econometric analysis yields various outputs, from coefficient estimates to statistical significance levels. Understanding these outputs is crucial for conveying findings effectively. - **Coefficients**: Each coefficient indicates the relationship between the dependent variable and predictors. A positive coefficient highlights a direct relationship, while a negative coefficient suggests the opposite. - **Statistical Significance**: P-values are used to assess statistical significance. Typically, a p-value below 0.05 indicates a statistically significant relationship, allowing you to confidently infer conclusions. - **Goodness-of-Fit Statistics**: Metrics like R-squared help evaluate the model's explanatory power. A higher R-squared indicates a better fit between your data and the econometric model. Understanding these components will enable researchers to translate their quantitative findings into actionable insights effectively. # Avoiding Common Pitfalls in Econometric Research. While econometrics offers powerful analytical techniques, it’s also fraught with potential pitfalls. Here are some common issues to be aware of: - **Omitted Variable Bias**: Failing to include relevant variables can lead to misleading results. Always consider variables that might influence your dependent variable. - **Endogeneity**: If an independent variable is correlated with the error term, it may result in biased estimates. Use instrumental variables to address this. - **Multicollinearity**: High correlation among independent variables can inflate standard errors. Conduct correlation tests during your data exploration phase to identify this. Being aware of these pitfalls will improve your econometric research's credibility and reliability. # Conclusion. In conclusion, utilizing econometrics in research can significantly enhance your understanding of economic relationships and inform effective decision-making. By mastering the foundational concepts, ensuring quality data, selecting appropriate models, interpreting results accurately, and avoiding common pitfalls, researchers can leverage econometrics to produce insightful and impactful findings. Whether you are embarking on an academic endeavor or a practical application, these guidelines will help you navigate the complex world of econometrics successfully. Embrace the journey as you contribute to the ongoing discourse in the field of economics! .